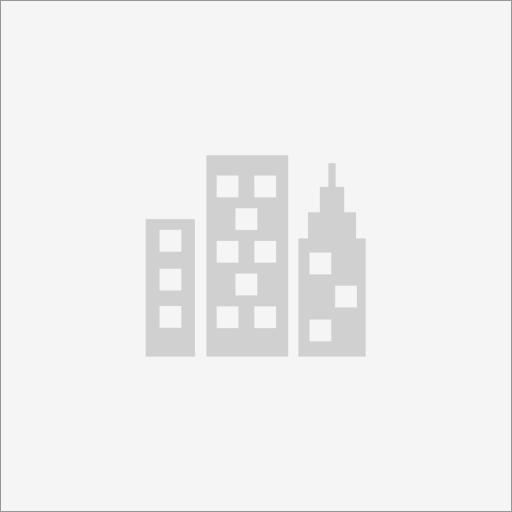
Université d'Angers
vacanciesin.eu
13 Feb 2024
Job Information
- Organisation/Company
- Université d’Angers
- Research Field
- Engineering
Mathematics
Computer science » Database management - Researcher Profile
- Recognised Researcher (R2)
Leading Researcher (R4)
First Stage Researcher (R1)
Established Researcher (R3) - Country
- France
- Application Deadline
- 11 Mar 2024 – 22:00 (UTC)
- Type of Contract
- Temporary
- Job Status
- Full-time
- Is the job funded through the EU Research Framework Programme?
- Not funded by an EU programme
- Is the Job related to staff position within a Research Infrastructure?
- No
Offer Description
Industry 4.0 should be seen today as a driver for rethinking conventional approaches with an openness to new paradigms, such as better utilization of data for decision support while reinforcing a holistic view of industrial tool management or control. In this discussion, we will address three challenges that maintenance, as an integrated activity within the overall production management process, must meet.
The first challenge is introduced as a thought and presented as one of the perspectives of this thesis work. The management of industrial systems, among others, is currently being influenced by the introduction of new digital evaluation and simulation tools, such as the Digital Twin (DT) and neural networks, e.g., for decision support. We will not revisit the definition of this DT here (see, for example, [1]). Studies of practical implementations of DT for maintenance in industrial environments have pointed out both advantages and development opportunities using DT for condition monitoring [2]. The study is a good basis for continued research into the use of DT. So far, the optimization aspect of maintenance planning has not been very well addressed. Carrying out maintenance at the right time, i.e. when there is a real need for maintenance, is an important aspect. It is also possible to reverse the optimization so that we control production equipment so that maintenance needs arise when a pre-planned maintenance stop occurs. What we call degradation aware control [3, 4]. The question asked here is therefore the use of a DT in the maintenance planning process.
The second challenge aims to improve cooperation between different enterprise processes, primarily within the framework of this work, between the production management and maintenance processes. Traditionally, in production problems, maintenance is considered a capacity-consuming activity. Maintenance planning then consists of determining the best compromise between minimizing production disruptions and ensuring reliable performance at the lowest cost. When maintenance models are solely based on lifetime distributions, so-called block or age-based policies can be used to define periodicity constraints that facilitate capacity reservation, [5, 6]. However, in the pursuit of performance and the use of associated monitoring systems, state-based approaches, where the maintenance decision is defined based on an estimated state, [7], could be integrated. State or prognostic indicators such as RUL (Residual Useful Lifetime) are then used to define the optimality structures of decision policies, [8]. This type of approach clearly serves as the basis for defining predictive maintenance, which is today one of the main motivations for industrial engineering research within the framework of Industry 4.0. One of the main challenges to ensure their integration is to consider, in the production model, this dynamic aspect of maintenance and thus address the absence of pre-established planning, [5, 9]. Moreover, the maintenance decision, which is usually optimized based on reliability characteristics related to age, should be adjusted according to the production context. These last two observations lead us to believe in the complexity of the joint optimization of plans for production and maintenance.
Given these observations, the thesis project will address the issue of integrating new maintenance approaches and their evolution towards prescriptive policies for better consideration in the context of tactical production planning. Prescriptive maintenance will be defined as the set of decisions that are both related to maintenance optimization (thresholds, periodicities, etc.) and operational constraints to ensure their effective implementation. It is thus seen as the highest level of maturity and complexity of knowledge-based maintenance (KBM), [10]. It can be seen as one of the levers for managing interactions with other processes such as quality management and production planning, [11, 12], and more recently [13].
The third challenge that this thesis project will address is related to performance indicators related to carbon emission reduction issues in the context of prescriptive maintenance. It should be noted that, by their very nature, maintenance objectives are clearly related to these aspects, but in a conventional model development framework, they are often too summarized to its economic dimension. More recently, we have seen the development of the concept of energy-based maintenance optimization, which aims to optimize maintenance activities to reduce energy consumption and improve the energy efficiency of systems. Its main and virtuous objective is to minimize energy waste, reduce operational costs, and promote sustainability by ensuring that equipment and systems operate at their highest level of energy efficiency.
Here too, prescriptive maintenance seems to us to be a promising approach to effective and rational energy management. In our vision, it should allow for the joint optimization of maintenance intervals based on a health indicator that takes into account current energy efficiency and usage prescriptions or “mission profiles” associated with consumption and energy efficiency predictions. For example, a prescription could result in a reduction in the productivity of a production system (reduction in output) to limit energy consumption, which is itself a function of its degradation level.
To address the challenges mentioned above, the doctoral candidate will first need to establish the positioning of their future contributions in the field of using physics-based approaches for the simulation and performance evaluation of complex systems in a maintenance context. They will need to demonstrate the benefits and challenges associated with prescriptive maintenance through case studies and analyze its potential in the context of energy-based maintenance optimization. Based on this in-depth literature review, the research program will be defined. The doctoral candidate can build upon preliminary work undertaken by the supervising team [14, 15] and [2-5], and in connection with the research projects they are conducting [14], e.g.
Bibliography
[1] Coupry, C.; Noblecourt, S.; Richard, P.; Baudry, D.; Bigaud, D. (2021). BIM-Based Digital Twin and XR Devices to Improve Maintenance Procedures in Smart Buildings: A Literature Review. Appl. Sci., 11, 6810. https://doi.org/10.3390/app11156810
[2] Hassan, M., Svadling, M., and Björsell, N., (2023) Experience from implementing digital twins for maintenance in industrial processes,, J. Intell. Manuf., 2023, doi: 10.1007/s10845-023-02078-4.
[3] Hosseinzadeh Dadash, A. and Björsell, N., (2023) Optimal Degradation-Aware Control Using Process-Controlled Sparse Bayesian Learning, Processes
[4] Björsell, N. and Hosseinzadeh Dadash, A.(2021) Finite Horizon Degradation Control of Complex Interconnected Systems, 17th IFAC Symposium on Information Control Problems in Manufacturing, INCOM, 2021.
[5] Lemoine, D., Castanier, B. (2021). Tactical Planning and Predictive Maintenance: Towards an Integrated Model Based on espilon-reliability. IFIP APMS2021: Advances in Production Management Systems, Sep 2021, Nantes, France. pp.92-101, 〈10.1007/978-3-030-85874-2_10〉.
[6] Pandey, D., Kulkarny, M. S., Vrat, P., (2011) A methodology for joint optimization for maintenance planning, process quality and production scheduling. Computers & Industrial Engineering, 61(4), 1098-1106
[7] Jafari, L., Makis, V. (2015). Joint optimal lot sizing and preventive maintenance policy for a production facility subject to condition monitoring. International Journal of Production Economics, 169(C), 156-168
[8] Lee, J., Ni, J., Djurdjanovic, D., Qiu, H., Liao, H. (2006). Intelligent prognostics tools and e-maintenance. Computers in Industry, 57(6), 476-489
[9] Huyn, K.T., Barros, A., Bérenguer, C. Castro, I. A periodic inspection and replacement policy for systems subject to competing failure modes due to degradation and traumatic events. Reliability Engineering and System Safety, Elsevier, 2011, 96 (4), pp.497-508.
[10] Ansari, F., Glawar, R., Sihn, W. (2017). Prescriptive Maintenance of CPPS by Integrating Multi-modal Data with Dynamic Bayesian Networks. In: Machine Learning for Cyber Physical Systems, Publisher: Springer. 1-8.
[11] Colledani, M., Tolio, T., Fischer, A., Iung, B., Lanza, G., Schmitt R., Vancza J. (2014). Design and management of manufacturing systems for production quality. CIRP Annals-Manufacturing Technology, 63(2), 773-796.
[12] El Maraghy, W., El Maraghy, H., Tomiyama, T., Monostori L. (2012). Complexity in engineering design and manufacturing. CIRP Annals-Manufacturing Technology, 61(2), 793-814
[13] Majdouline, I., Dellagi, S., Mifdal, L., Kibbou, E. M., Moufki, A. (2021). Integrated production-maintenance strategy considering quality constraints in dry machining. International Journal of Production Research, DOI: 10.1080/00207543.2021.1905193
[14] Esposito, N. Extension of the gamma process and its application to prescriptive and condition-based maintenance under imperfect information. PhD thesis, Université d’Angers (France) and Università degli studi di Napoli Federico II (Defended in December, 2023)
[14] Esposito, N., Castanier, B., Giorgio, M. A Prescriptive Maintenance Policy for a Gamma Deteriorating Unit. In the proceedings of 32nd European Safety and Reliability Conference (ESREL 2022). 2022
[15] Planning, Scheduling and Maintenance for Integrated and Robust Production (POMPIER) – ANR research project (in French) – February 2024 to February 2028
Funding category: Autre financement public
Co-financement France Suède
PHD title: Doctorat en Sciences Pour l’Ingénieur – Option Génie Industriel
PHD Country: France
Requirements
Specific Requirements
The applicant must have a Master degree or equivalent in Industrial Engineering, Applied Mathematics (Data Science) and has to prove his/her skills in computation. Communication and project management are important. The language of the PhD program will be English, Swedish or French are welcome.
Additional Information
Work Location(s)
- Number of offers available
- 1
- Company/Institute
- Université d’Angers
- Country
- France
- City
- Angers
- Geofield
Where to apply
- Website
- https://www.abg.asso.fr/fr/candidatOffres/show/id_offre/120239
Contact
- Website
- https://laris.univ-angers.fr/fr/home.html
STATUS: EXPIRED
View or Apply
To help us track our recruitment effort, please indicate in your cover//motivation letter where (vacanciesin.eu) you saw this job posting.